AI-Driven Drug Discovery: Target-Specific Molecule Generation for Cancer Pathways
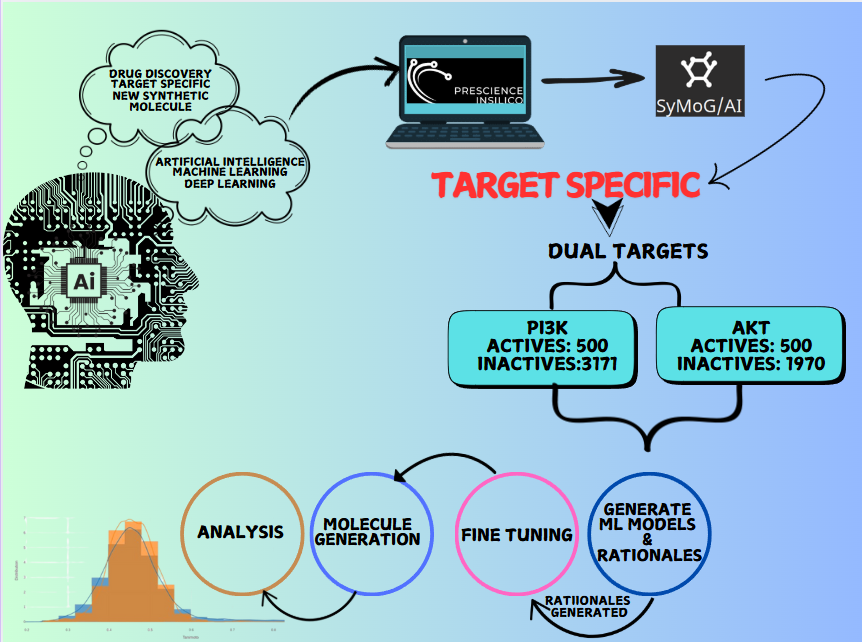
The Target Specific module within the SyMoG/AI application represents a groundbreaking approach to synthetic molecule generation tailored to specific biological targets, focusing on drug discovery for cancer-related pathways. Leveraging machine learning models trained on active and inactive molecular representations (SMILES), the module facilitates identifying and creating molecules with desired properties. Cancer remains one of the most formidable challenges in modern medicine, driven by diverse genetic and molecular factors that fuel tumor development, progression, and resistance to treatment. The PI3K/Akt signaling plays a pivotal role among the various molecular pathways implicated in cancer, particularly in brain and central nervous system tumors and endocrine, respiratory, breast, and gynecological cancers. This pathway regulates cell growth, survival, and metabolism, and its dysregulation is a key contributor to oncogenesis and therapy resistance. Further, hyperactivation of this pathway has been linked to multidrug resistance (MDR) by upregulating efflux pumps like ABC transporters and inhibiting chemotherapy-induced apoptosis.
Target-specific is an AI-driven application that transforms drug discovery by generating novel synthetic molecules tailored to meet intricate chemical property requirements. Using active and inactive SMILES strings, SyMoG/AI enables the exploration of uncharted chemical spaces without relying on predefined target structures. This efficiency reduces the time and cost of discovering high-quality candidate molecules. This case study explores the capabilities of SyMoG/AI, a cutting-edge platform that uses machine learning (ML) models trained on active and inactive SMILES (Simplified Molecular Input Line Entry System) representations of PI3K and Akt targets. The active and inactive SMILES were generated using ChemIn and filtered based on IC50 activity value. Users can include multi-targets for studying multitarget pathways. To facilitate this, an intuitive feature allows researchers to analyze multiple targets and design molecules with activity across all of them.
Generating Model and Rationales
The active and inactive SMILES representations are used for training models because they provide labeled data that helps the model learn the relationship between molecular structures and their corresponding properties. After training a model, its performance is assessed using metrics like the Model Score to evaluate how well it predicts desired properties and Root Mean Square Error (RMSE) to measure the average deviation of predictions from actual values. A lower RMSE and a higher model score indicate better predictive accuracy. This evaluation ensures the model is reliable, generalizes well to unseen data, and is suitable for downstream tasks like generating molecules effectively. The software offers an intuitive feature for generating rationales, which are key substructures in molecules responsible for specific properties. To generate rationales, click the “Generate Rationale” button. Once complete, click the download icon to save the results, including the identified substructures linked to the target properties. Rationales are key substructures within molecules that contribute to specific properties. For the Akt and PI3K ML models, the software generated a total of 5,637 rationales, of which 258 rationales were filtered. This includes 143 rationales for the PI3K target and 115 rationales for the AKT target. In molecule generation, these rationales serve as building blocks. After identifying the rationales, the software uses them to create new molecules by combining and expanding these substructures. This helps generate compounds with the desired properties while maintaining chemical validity. Essentially, rationales guide the design of new molecules, ensuring they meet target property constraints.
Finetuning
Fine-tuning is the process of further training a pre-trained model to improve its ability to generate molecules with specific properties. During fine-tuning, users set epochs (how often the model processes the data) and decode numbers (how many molecules structures the model generates per input). Here, we used 5 epochs and 100 decodes to fine-tune the model. This process merges identified rationales and key substructures responsible for certain properties into building blocks for new molecules. The model is refined to combine these rationales to generate molecules with desired properties, such as activity or drug-likeness. After fine-tuning, the model becomes better at generating valid and optimized molecules that meet the specified constraints, improving the accuracy and diversity of the compounds. Since fine-tuning is computationally intensive, it is recommended that a GPU-enabled machine be used.
Molecule Generation and Evaluation
After fine-tuning, we used the "Molecule Generation" button to create 299 novel molecules with desired properties. These molecules were evaluated based on their QA (Quality Assurance), which measures how well they meet chemical and biological properties, and SA (Synthetic Accessibility), which indicates how easily they can be synthesized (lower SA values mean easier synthesis). The visualizer displays these values and a performance score (ranging from 0 to 1) to assess how well the molecules perform against two targets, with higher scores indicating better results. The Fraction of Actives is 0.0034, indicating that a small percentage of the generated molecules are predicted to exhibit the desired biological or chemical activity. Similarly, the Fraction of Unique Actives, also 0.0034, shows that only a small proportion of active molecules have unique structures, ensuring limited diversity among the active compounds. The Active Reference Count refers to the number of known active compounds used by the model as a reference to guide the generation of new molecules. The novelty score is 1.0, indicating that all the generated molecules are novel and have not been seen in the reference database, ensuring the uniqueness of the generated compounds. These values highlight the challenges in achieving diversity and activity in molecule generation while demonstrating that the generated molecules are innovative and distinct. These 299 generated molecules come with detailed physicochemical properties, aiding in further analysis and optimization.
Analysis
The Analysis module in the software enables users to manage, analyze, and export molecular data efficiently. It presents a table interface that supports datasets ranging from 10 to 1000 molecules, displaying both input and output molecules along with their chemical properties. Users can filter molecules based on key criteria such as drug-likeness, Lipinski's Rule of Five, and synthesizability. The dataset includes properties like SMILES (molecular structure), synthesizability score, LogP (lipophilicity), solubility, drug-likeness score, molecular weight, hydrogen bond donors and acceptors, and halogens. To shortlist potential drug candidates, the following criteria are used: synthesizability scores below 3.5, molecules that adhere to Lipinski's Rule of Five (LogP ≤ 5, molecular weight ≤ 500, hydrogen bond donors ≤ 5, and hydrogen bond acceptors ≤ 10), and drug-likeness scores between 0.5 and 1. After applying these filters, 11 molecules are identified as strong candidates for further in silico studies, such as docking and molecular dynamics simulations in the XESS application within the PrinS3 platform. For further details, reach out to us at support@prescience.in